Michael Gall resumes & CV records
Resumes
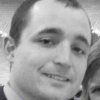
Prevention Educator At Safe Connections
Position:
Prevention Educator at Safe Connections
Location:
Saint Louis, Missouri
Industry:
Civic & Social Organization
Work:
Safe Connections
since Jul 2012
Prevention Educator Meds & Food for Kids Aug 2010 - Jan 2012
Communications Specialist Washington University in St. Louis - St. Louis Aug 2011 - Dec 2011
Center for Mental Health Services Research RA Other Places Publishing Dec 2009 - Aug 2010
Contributing Writer Peace Corps - Federated States of Micronesia Aug 2007 - Nov 2009
ESL Instructor / Community Organizer Mercy Franciscan at St. John - Cincinnati Area Aug 2008 - May 2009
Practicum Student
Prevention Educator Meds & Food for Kids Aug 2010 - Jan 2012
Communications Specialist Washington University in St. Louis - St. Louis Aug 2011 - Dec 2011
Center for Mental Health Services Research RA Other Places Publishing Dec 2009 - Aug 2010
Contributing Writer Peace Corps - Federated States of Micronesia Aug 2007 - Nov 2009
ESL Instructor / Community Organizer Mercy Franciscan at St. John - Cincinnati Area Aug 2008 - May 2009
Practicum Student
Education:
Washington University in St. Louis 2010 - 2011
Master of Social Work (MSW) Xavier University 2003 - 2007
Bachelor, Social Work
Master of Social Work (MSW) Xavier University 2003 - 2007
Bachelor, Social Work
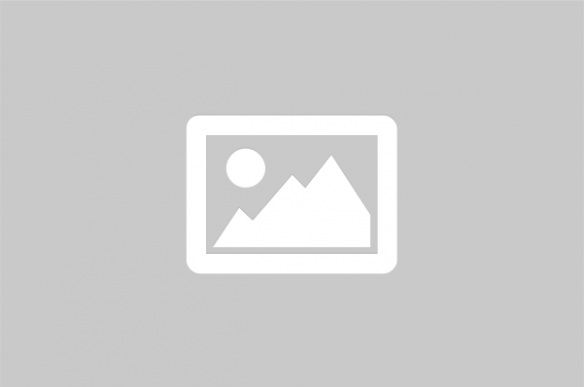
Michael Gall
Location:
United States
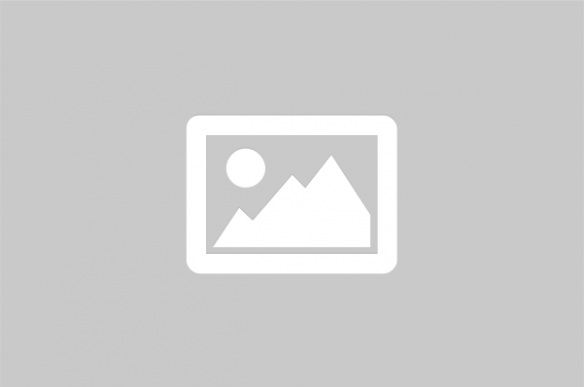
Michael Gall
Location:
United States
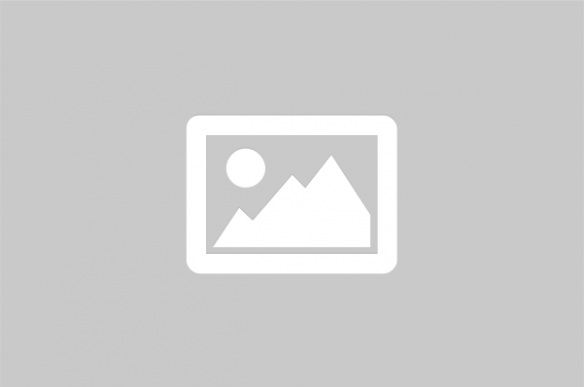
Michael Gall
Location:
United States