Shen Huang resumes & CV records
Resumes
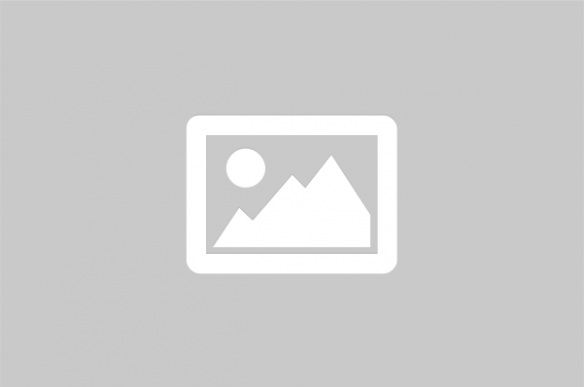
Accelerated Value Specialist
Accelerated Value Specialist Ibm 2001 - Dec 2005
Advisory Software Engineer at Ibm Corp Informix Software Jan 1998 - Jun 2001
Advisory Sofware Engineer Informix Software Jan 1998 - Apr 2001
Software Engineer University of Pennsylvania Jan 1993 - Dec 1997
Business Analyst
Master of Science, Masters, Computer Science Fudan University 1978 - 1982
Bachelors, Bachelor of Science, Biophysics
Mandarin
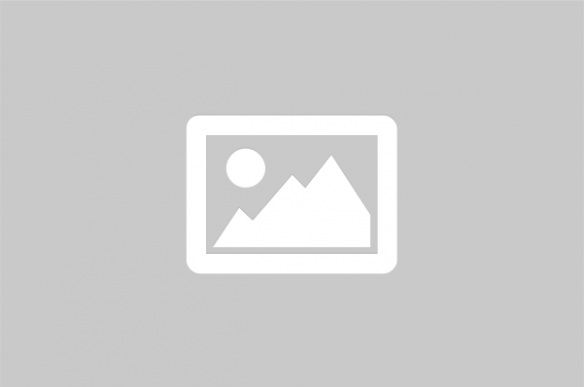